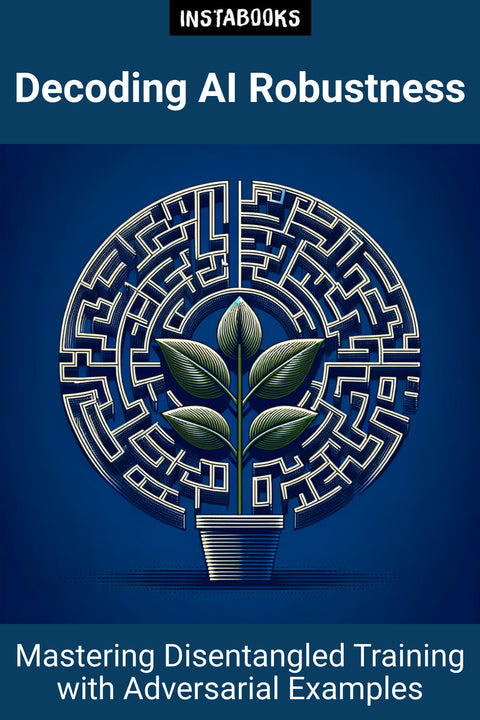
Instabooks AI (AI Author)
Decoding AI Robustness
Mastering Disentangled Training with Adversarial Examples
Premium AI Book (PDF/ePub) - 200+ pages
An Intriguing Journey into AI's Future
In Decoding AI Robustness: Mastering Disentangled Training with Adversarial Examples, embark on a fascinating exploration of how modern AI systems are fortified against adversarial challenges. At the heart of this book is a critical examination of how disentangled training and adversarial examples play a pivotal role in building small-footprint, robust keyword spotting models. The narrative is crafted to be both enlightening and practical, providing insights that are essential for AI enthusiasts, researchers, and practitioners.
Exploring Core Concepts
The book delves deep into the science of adversarial examples and disentangled representations, uncovering the secrets of these ingenious inputs designed to fool AI models. These ideas are elaborated with clarity and depth, covering the essentials of adversarial training, a method used to strengthen model robustness by exposing them to cleverly crafted adversarial examples. Furthermore, readers will discover the exciting potential of Deep Robust Representation Disentanglement Networks (DRRDN) in enhancing AI resilience.
Innovative Defense Mechanisms
Learn how to employ advanced defense mechanisms like DRRDN that disentangle robust parts of data from vulnerable elements, ensuring AI models are versatile and reliable. The book offers a detailed guide to understand how data-specific disentanglement adds another layer of defense in specific applications such as keyword spotting, which demands precise and resilient models in the face of adversarial threats.
Insights into Practical Applications
Beyond theory, this book transitions into practical scenarios showing how disentangled training can fortify keyword spotting. It uncovers methodological approaches for applying autoencoders with disentangled representations to meticulously detect adversarial examples, thereby refining the models’ abilities in real-world applications. These insights empower developers to build more resilient AI systems that can withstand real-world challenges.
Connecting Theory with Practice
This comprehensive guide is supported by exhaustive research and insights from leading academic papers and innovative methodologies, equipping readers with the knowledge to merge theory with practical application. Whether you're aiming to enhance your understanding or apply these concepts, this book is an essential resource for navigating the challenges and opportunities in the domain of robust AI models.
Table of Contents
1. Understanding Adversarial Examples- Impact on AI Models
- Creation and Characteristics
- Real-World Implications
2. The Science of Disentangled Representations
- Fundamental Concepts
- Applications in AI
- Challenges and Innovations
3. Adversarial Training Unveiled
- Balancing Accuracy and Robustness
- Techniques and Strategies
- Future Prospects
4. Introducing DRRDN
- Conceptual Framework
- Implementation Details
- Case Studies
5. Data-Specific Disentanglement Strategies
- Customizing for Datasets
- Advantages and Limitations
- Industry Applications
6. Enhancing Robustness in Keyword Spotting
- Techniques and Tools
- Practical Implementation
- Evaluating Effectiveness
7. Detecting Adversarial Examples
- Behavior Analysis
- Detection Methods
- Improving Model Defenses
8. Methodological Approaches
- Algorithmic Innovations
- Case Studies
- Integration with Existing Systems
9. Practical Insights and Applications
- Real-World Case Studies
- Strategies for Implementation
- Success Stories
10. Challenges and Opportunities Ahead
- Technological Challenges
- Exploring New Frontiers
- The Future of AI Robustness
11. Bridging Theory and Practice
- Educational Insights
- Hands-On Tutorials
- Learning Pathways for Practitioners
12. Crafting the Future of AI
- Innovation in AI Development
- Ethical Considerations
- Global Impact and Opportunities
Target Audience
This book is designed for AI researchers, machine learning practitioners, and enthusiasts who seek to deepen their understanding of model robustness through disentangled training and adversarial examples.
Key Takeaways
- Comprehensive insights into disentangled training and adversarial examples.
- Understanding and implementing DRRDN to enhance model robustness.
- Practical applications in keyword spotting and defense mechanisms.
- Methodological approaches to detect adversarial examples.
- Insights into current challenges and future opportunities in AI robustness.
How This Book Was Generated
This book is the result of our advanced AI text generator, meticulously crafted to deliver not just information but meaningful insights. By leveraging our AI book generator, cutting-edge models, and real-time research, we ensure each page reflects the most current and reliable knowledge. Our AI processes vast data with unmatched precision, producing over 200 pages of coherent, authoritative content. This isn’t just a collection of facts—it’s a thoughtfully crafted narrative, shaped by our technology, that engages the mind and resonates with the reader, offering a deep, trustworthy exploration of the subject.
Satisfaction Guaranteed: Try It Risk-Free
We invite you to try it out for yourself, backed by our no-questions-asked money-back guarantee. If you're not completely satisfied, we'll refund your purchase—no strings attached.